If A makes B more likely then B makes A more likely" Announcing the arrival of Valued Associate #679: Cesar Manara Planned maintenance scheduled April 17/18, 2019 at 00:00UTC (8:00pm US/Eastern)What is a very intuitive way to teach the Bayes formula to undergraduates?How would you explain covariance to someone who understands only the mean?Why does “explaining away” make intuitive sense?What is the intuition behind (M)ANCOVA and when/why should one use it?An example of r.v.s such that their distribution has more (conditional) independencies than their directed graphical modelIntuition for “weights” in simple linear regressionIntuitive explanation of the F-statistic formula?the intuition behind that the variance of increment for Brownian Motion is time intervalWhy is it that when the score variance gets LARGER we get MORE confident about the MLE estimate?Intuition behind infinite CRLBWhy do parametric models learn more slowly by design?
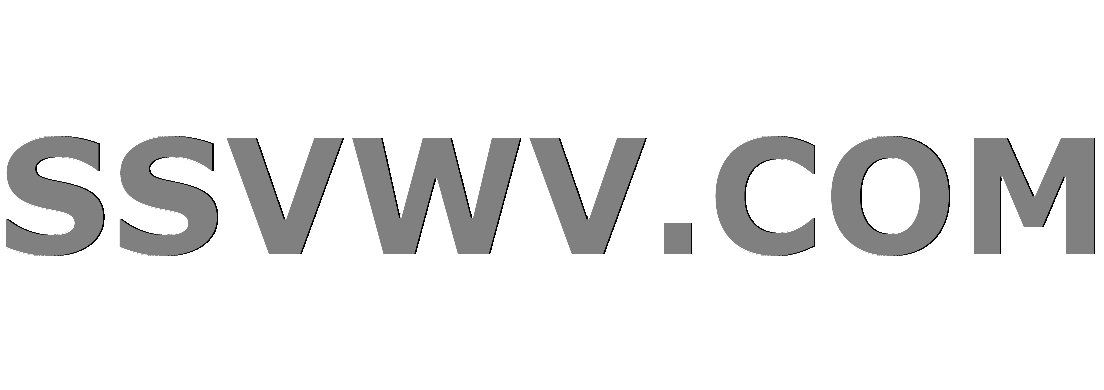
Multi tool use
Single author papers against my advisor's will?
Two different pronunciation of "понял"
Mortgage adviser recommends a longer term than necessary combined with overpayments
How to market an anarchic city as a tourism spot to people living in civilized areas?
Do working physicists consider Newtonian mechanics to be "falsified"?
Jazz greats knew nothing of modes. Why are they used to improvise on standards?
Is it possible to ask for a hotel room without minibar/extra services?
Slither Like a Snake
Is dark matter really a meaningful hypothesis?
What LEGO pieces have "real-world" functionality?
Is above average number of years spent on PhD considered a red flag in future academia or industry positions?
Why does tar appear to skip file contents when output file is /dev/null?
How do you clear the ApexPages.getMessages() collection in a test?
Active filter with series inductor and resistor - do these exist?
Passing functions in C++
Cold is to Refrigerator as warm is to?
Why is "Captain Marvel" translated as male in Portugal?
If I can make up priors, why can't I make up posteriors?
How to say 'striped' in Latin
Why don't the Weasley twins use magic outside of school if the Trace can only find the location of spells cast?
When communicating altitude with a '9' in it, should it be pronounced "nine hundred" or "niner hundred"?
Typsetting diagram chases (with TikZ?)
Unexpected result with right shift after bitwise negation
How to rotate it perfectly?
If A makes B more likely then B makes A more likely"
Announcing the arrival of Valued Associate #679: Cesar Manara
Planned maintenance scheduled April 17/18, 2019 at 00:00UTC (8:00pm US/Eastern)What is a very intuitive way to teach the Bayes formula to undergraduates?How would you explain covariance to someone who understands only the mean?Why does “explaining away” make intuitive sense?What is the intuition behind (M)ANCOVA and when/why should one use it?An example of r.v.s such that their distribution has more (conditional) independencies than their directed graphical modelIntuition for “weights” in simple linear regressionIntuitive explanation of the F-statistic formula?the intuition behind that the variance of increment for Brownian Motion is time intervalWhy is it that when the score variance gets LARGER we get MORE confident about the MLE estimate?Intuition behind infinite CRLBWhy do parametric models learn more slowly by design?
.everyoneloves__top-leaderboard:empty,.everyoneloves__mid-leaderboard:empty,.everyoneloves__bot-mid-leaderboard:empty margin-bottom:0;
$begingroup$
I am trying to get a clearer intuition behind: "If $A$ makes $B$ more likely then $B$ makes $A$ more likely" i.e
Let $n(S)$ denote the size of the space in which $A$ and $B$ are, then
Claim: $P(B|A)>P(B)$ so $n(AB)/n(A) > n(B)/n(S)$
so $n(AB)/n(B) > n(A)/n(S)$
which is $P(A|B)>P(A)$
I understand the math, but why does this make intuitive sense?
probability inference conditional-probability intuition association-measure
$endgroup$
add a comment |
$begingroup$
I am trying to get a clearer intuition behind: "If $A$ makes $B$ more likely then $B$ makes $A$ more likely" i.e
Let $n(S)$ denote the size of the space in which $A$ and $B$ are, then
Claim: $P(B|A)>P(B)$ so $n(AB)/n(A) > n(B)/n(S)$
so $n(AB)/n(B) > n(A)/n(S)$
which is $P(A|B)>P(A)$
I understand the math, but why does this make intuitive sense?
probability inference conditional-probability intuition association-measure
$endgroup$
add a comment |
$begingroup$
I am trying to get a clearer intuition behind: "If $A$ makes $B$ more likely then $B$ makes $A$ more likely" i.e
Let $n(S)$ denote the size of the space in which $A$ and $B$ are, then
Claim: $P(B|A)>P(B)$ so $n(AB)/n(A) > n(B)/n(S)$
so $n(AB)/n(B) > n(A)/n(S)$
which is $P(A|B)>P(A)$
I understand the math, but why does this make intuitive sense?
probability inference conditional-probability intuition association-measure
$endgroup$
I am trying to get a clearer intuition behind: "If $A$ makes $B$ more likely then $B$ makes $A$ more likely" i.e
Let $n(S)$ denote the size of the space in which $A$ and $B$ are, then
Claim: $P(B|A)>P(B)$ so $n(AB)/n(A) > n(B)/n(S)$
so $n(AB)/n(B) > n(A)/n(S)$
which is $P(A|B)>P(A)$
I understand the math, but why does this make intuitive sense?
probability inference conditional-probability intuition association-measure
probability inference conditional-probability intuition association-measure
edited 6 hours ago


Aaron Hall
251214
251214
asked yesterday
Rahul DeoraRahul Deora
1108
1108
add a comment |
add a comment |
10 Answers
10
active
oldest
votes
$begingroup$
To add on the answer by @Dasherman: What can it mean to say that two events are related, or maybe associated or correlated? Maybe we could for a definition compare the joint probability (Assuming $DeclareMathOperatorPmathbbP P(A)>0, P(B)>0$):
$$
eta(A,B)=fracP(A cap B)P(A) P(B)
$$
so if $eta$ is larger than one, $A$ and $B$ occurs together more often than under independence. Then we can say that $A$ and $B$ are positively related.
But now, using the definition of conditional probability, $fracP(A cap B)P(A) P(B)>1$ is an easy consequence of $P(B mid A) > P(B)$. But $fracP(A cap B)P(A) P(B)$ is completely symmetric in $A$ and $B$ (interchanging all occurrences of the symbol $A$ with $B$ and vice versa) leaves the same formulas, so is also equivalent with $P(A mid B) > P(A)$. That gives the result. So the intuition you ask for is that $eta(A,B)$ is symmetric in $A$ and $B$.
The answer by @gunes gave a practical example, and it is easy to make others the same way.
$endgroup$
add a comment |
$begingroup$
I think another mathematical way of putting it may help. Consider the claim in the context of Bayes' rule:
Claim: if $P(B|A)>P(B)$ then $P(A|B) > P(A)$
Bayes' rule:
$$ P(A mid B) = fracP(B mid A) , P(A)P(B) $$
assuming $P(B)$ nonzero. Thus
$$fracB)P(A) = fracP(BP(B)$$
If $P(B|A)>P(B)$, then $fracP(BP(B) > 1$.
Then $fracB)P(A) > 1$, and so $P(A|B) > P(A)$.
This proves the claim and an even stronger conclusion - that the respective proportions of the likelihoods must be equal.
$endgroup$
add a comment |
$begingroup$
Well, I don't like the word "makes" in the question. That implies some sort of causality and causality usually doesn't reverse.
But you asked for intuition. So, I'd think about some examples, because that seems to spark intuition. Choose one you like:
If a person is a woman, it is more likely that the person voted for a Democrat.
If a person voted for a Democrat, it is more likely that the person is a woman.
If a man is a professional basketball center, it is more likely that he is over 2 meters tall.
If a man is over 2 meters tall, it is more likely that he is a basketball center.
If it is over 40 degrees Celsius, it is more likely that there will be a blackout.
If there has been a blackout, it is more likely that it is over 40 degrees.
And so on.
$endgroup$
2
$begingroup$
That's not about probability. That's about 1 to 1 relationships.
$endgroup$
– Peter Flom♦
16 hours ago
2
$begingroup$
@jww Imagine the statement "if it is raining, the street is wet" (and suppose that's a valid implication for the moment, while the converse is not). Now take a large number of "samples" in different times and places, where you record whether it's raining and whether the street is wet. The street will be wet in more of the samples where it's raining than the samples where it's not; but also, it will be raining in more of the samples where the street is wet than the samples where the street is dry. That's probability.
$endgroup$
– hobbs
14 hours ago
1
$begingroup$
Both phenomena are caused by the same implication; the implication only works one way, but observing the consequent makes it more likely that you're looking at a sample where the antecedent is true.
$endgroup$
– hobbs
14 hours ago
1
$begingroup$
@Barmar Sorry, but that partly demonstrates the correctness of my logic. Because say 36/25,000 is a whole lot higher than 1/150,000,000.
$endgroup$
– Peter Flom♦
9 hours ago
1
$begingroup$
More likely than someone who is less than 2 meters tall.
$endgroup$
– Peter Flom♦
9 hours ago
|
show 4 more comments
$begingroup$
By way of intuition, real world examples such as Peter Flom gives are most helpful for some people. The other thing that commonly helps people is pictures. So, to cover most bases, let's have some pictures.
What we have here are two very basic diagrams showing probabilities. The first shows two independent predicates I'll call Red and Plain. It is clear that they are independent because the lines line up. The proportion of plain area that is red is the same as the proportion of stripy area that is red and is also the same as the total proportion that is red.
In the second image, we have non-independent distributions. Specifically, we have moved some of the stripy red area into the plain area without changing the fact that it is red. Clearly then, being red makes being plain more likely.
Meanwhile, have a look at the plain side of that image. Clearly the proportion of the plain region that is red is greater than the proportion of the whole image that is red. That is because the plain region has been given a bunch more area and all of it is red.
So, red makes plain more likely, and plain makes red more likely.
What's actually happening here? A is evidence for B (that is, A makes B more likely) when the area that contains both A and B is bigger than would be predicted if they were independent. Because the intersection between A and B is the same as the intersection between B and A, that also implies that B is evidence for A.
One note of caution: although the argument above seems very symmetrical, it may not be the case that the strength of evidence in both directions is equal. For example, consider this third image.
Here the same thing has happened: plain red has eaten up territory previously belonging to stripy red. In fact, it has completely finished the job!
Note that the point being red outright guarantees plainness because there are no stripy red regions left. However a point being plain has not guaranteed redness, because there are still green regions left. Nevertheless, a point in the box being plain increases the chance that it is red, and a point being red increases the chance that it is plain. Both directions imply more likely, just not by the same amount.
New contributor
Josiah is a new contributor to this site. Take care in asking for clarification, commenting, and answering.
Check out our Code of Conduct.
$endgroup$
add a comment |
$begingroup$
If A makes B more likely, this means the events are somehow related. This relation works both ways.
If A makes B more likely, this means that A and B tend to happen together. This then means that B also makes A more likely.
$endgroup$
1
$begingroup$
This perhaps could use some expansion? Without a definition of related it is a bit empty.
$endgroup$
– mdewey
19 hours ago
2
$begingroup$
I was trying to stay away from anything rigorous, since OP asked for an intuitive explanation. You are right that it is quite empty as it is now, but I'm not sure how to expand it in an intuitive way. I have added an attempt.
$endgroup$
– Dasherman
18 hours ago
add a comment |
$begingroup$
If A makes B more likely, A has crucial information that B can infer about itself. Despite the fact that it might not contribute the same amount, that information is not lost the other way around. Eventually, we have two events that their occurrence support each other. I can’t seem to imagine a scenario where occurrence of A increases the likelihood of B, and occurrence of B decreases the likelihood of A. For example, if it rains, the floor will be wet with high probability, and if the floor is wet, it doesn’t mean that it rained but it doesn’t decrease the chances.
$endgroup$
add a comment |
$begingroup$
You are told that Sam is a woman and Kim is a man, and one of the two wears make-up and the other does not. Who of them would you guess wears make-up?
You are told that Sam wears make-up and Kim doesn't, and one of the two is a man and one is a woman. Who would you guess is the woman?
$endgroup$
$begingroup$
It is not so straightforward to connect this to the original problem. What exactly is event A and what is event B? Here it seems more like some comparison of probabilities. Event A is 'x is a women' (not A is the event 'x is a man'). And event B is 'x wears makeup'. But now we suddenly have a Sam and a Kim, where does that come from and should we use anything of information about the subjective masculinity or femininity of their names?
$endgroup$
– Martijn Weterings
11 hours ago
add a comment |
$begingroup$
You can make the math more intuitive by imagining a contingency table.
$beginarraycc
beginarraycc
&& A & lnot A & \
&a+b+c+d & a+c & b+d \hline
B& a+b& a & b \
lnot B & c+d& c & d \
endarray
endarray$
When $A$ and $B$ are independent then the joint probabilities are products of the marginal probabilities $$beginarraycc
beginarraycc
&& A & lnot A & \
&1 & x & 1-x \hline
B& y& a=xy & b=(1-x)y \
lnot B & 1-y& c=x (1-y) & d=(1-x)(1-y)\
endarray
endarray$$ In such case you would have similar marginal and conditional probabilities, e.g. $P (A) = P (A|B) $ and $P (B)=P (B|A) $.When there is no independence then you could see this as leaving the parameters $a,b,c,d $ the same (as products of the margins) but with just an adjustment by $pm z $ $$beginarraycc
beginarraycc
&& A & lnot A & \
&1 & x & 1-x \hline
B& y& a+z & b-z \
lnot B & 1-y& c-z & d+z\
endarray
endarray$$You could see this $z$ as breaking the equality of the marginal and conditional probabilities or breaking the relationship for the joint probabilities being products of the marginal probabilities.
Now, from this point of view (of breaking these equalities) you can see that this breaking happens in two ways both for $P(A|B) neq P(A)$ and $P(B|A) neq P(B)$. And the inequality will be for both cases $>$ when $z$ is positive and $<$ when $z $ is negative.
So you could see the connection $P(A|B) > P(A)$ then $P(B|A) > P(B)$ via the joint probability $P(B,A) > P (A) P (B) $.
If A and B often happen together (joint probability is higher then product of marginal probabilities) then observing the one will make the (conditional) probability of the other higher.
$endgroup$
add a comment |
$begingroup$
Suppose we denote the posterior-to-prior probability ratio of an event as:
$$Delta(A|B) equiv fracB)mathbbP(A)$$
Then an alternative expression of Bayes' theorem (see this related post) is:
$$Delta(A|B)
= fracB)mathbbP(A)
= fracmathbbP(A cap B)mathbbP(A) mathbbP(B)
= fracA)mathbbP(B)
= Delta(B|A).$$
The posterior-to-prior probability ratio tells us whether the argument event is made more or less likely by the occurrence of the conditioning event (and how much more or less likely). The above form of Bayes' theorem shows use that posterior-to-prior probability ratio is symmetric in the variables.$^dagger$ For example, if observing $B$ makes $A$ more likely than it was a priori, then observing $A$ makes $B$ more likely than it was a priori.
$^dagger$ Note that this is a probability rule, and so it should not be interpreted causally. This symmetry is true in a probabilistic sense for passive observation ---however, it is not true if you intervene in the system to change $A$ or $B$. In that latter case you would need to use causal operations (e.g., the $textdo$ operator) to find the effect of the change in the conditioning variable.
$endgroup$
add a comment |
$begingroup$
There is a confusion here between causation and correlation. So I'll give you an example where the exact opposite happens.
Some people are rich, some are poor. Some poor people are given benefits, which makes them less poor. But people who get benefits are still more likely to be poor, even with benefits.
If you are given benefits, that makes it more likely that you can afford cinema tickets. ("Makes it more likely" meaning causality). But if you can afford cinema tickets, that makes it less likely that you are among the people who are poor enough to get benefits, so if you can afford cinema tickets, you are less likely to get benefits.
$endgroup$
4
$begingroup$
This isn't an answer to the question. Interesting, but not an answer. In fact, it's talking about a different scenario; the reason the opposite happens is that it's using two different metrics that are named similarly (poor without benefits v.s. poor with benefits) and as such is a completely different scenario.
$endgroup$
– wizzwizz4
16 hours ago
add a comment |
Your Answer
StackExchange.ready(function()
var channelOptions =
tags: "".split(" "),
id: "65"
;
initTagRenderer("".split(" "), "".split(" "), channelOptions);
StackExchange.using("externalEditor", function()
// Have to fire editor after snippets, if snippets enabled
if (StackExchange.settings.snippets.snippetsEnabled)
StackExchange.using("snippets", function()
createEditor();
);
else
createEditor();
);
function createEditor()
StackExchange.prepareEditor(
heartbeatType: 'answer',
autoActivateHeartbeat: false,
convertImagesToLinks: false,
noModals: true,
showLowRepImageUploadWarning: true,
reputationToPostImages: null,
bindNavPrevention: true,
postfix: "",
imageUploader:
brandingHtml: "Powered by u003ca class="icon-imgur-white" href="https://imgur.com/"u003eu003c/au003e",
contentPolicyHtml: "User contributions licensed under u003ca href="https://creativecommons.org/licenses/by-sa/3.0/"u003ecc by-sa 3.0 with attribution requiredu003c/au003e u003ca href="https://stackoverflow.com/legal/content-policy"u003e(content policy)u003c/au003e",
allowUrls: true
,
onDemand: true,
discardSelector: ".discard-answer"
,immediatelyShowMarkdownHelp:true
);
);
Sign up or log in
StackExchange.ready(function ()
StackExchange.helpers.onClickDraftSave('#login-link');
);
Sign up using Google
Sign up using Facebook
Sign up using Email and Password
Post as a guest
Required, but never shown
StackExchange.ready(
function ()
StackExchange.openid.initPostLogin('.new-post-login', 'https%3a%2f%2fstats.stackexchange.com%2fquestions%2f402935%2fif-a-makes-b-more-likely-then-b-makes-a-more-likely%23new-answer', 'question_page');
);
Post as a guest
Required, but never shown
10 Answers
10
active
oldest
votes
10 Answers
10
active
oldest
votes
active
oldest
votes
active
oldest
votes
$begingroup$
To add on the answer by @Dasherman: What can it mean to say that two events are related, or maybe associated or correlated? Maybe we could for a definition compare the joint probability (Assuming $DeclareMathOperatorPmathbbP P(A)>0, P(B)>0$):
$$
eta(A,B)=fracP(A cap B)P(A) P(B)
$$
so if $eta$ is larger than one, $A$ and $B$ occurs together more often than under independence. Then we can say that $A$ and $B$ are positively related.
But now, using the definition of conditional probability, $fracP(A cap B)P(A) P(B)>1$ is an easy consequence of $P(B mid A) > P(B)$. But $fracP(A cap B)P(A) P(B)$ is completely symmetric in $A$ and $B$ (interchanging all occurrences of the symbol $A$ with $B$ and vice versa) leaves the same formulas, so is also equivalent with $P(A mid B) > P(A)$. That gives the result. So the intuition you ask for is that $eta(A,B)$ is symmetric in $A$ and $B$.
The answer by @gunes gave a practical example, and it is easy to make others the same way.
$endgroup$
add a comment |
$begingroup$
To add on the answer by @Dasherman: What can it mean to say that two events are related, or maybe associated or correlated? Maybe we could for a definition compare the joint probability (Assuming $DeclareMathOperatorPmathbbP P(A)>0, P(B)>0$):
$$
eta(A,B)=fracP(A cap B)P(A) P(B)
$$
so if $eta$ is larger than one, $A$ and $B$ occurs together more often than under independence. Then we can say that $A$ and $B$ are positively related.
But now, using the definition of conditional probability, $fracP(A cap B)P(A) P(B)>1$ is an easy consequence of $P(B mid A) > P(B)$. But $fracP(A cap B)P(A) P(B)$ is completely symmetric in $A$ and $B$ (interchanging all occurrences of the symbol $A$ with $B$ and vice versa) leaves the same formulas, so is also equivalent with $P(A mid B) > P(A)$. That gives the result. So the intuition you ask for is that $eta(A,B)$ is symmetric in $A$ and $B$.
The answer by @gunes gave a practical example, and it is easy to make others the same way.
$endgroup$
add a comment |
$begingroup$
To add on the answer by @Dasherman: What can it mean to say that two events are related, or maybe associated or correlated? Maybe we could for a definition compare the joint probability (Assuming $DeclareMathOperatorPmathbbP P(A)>0, P(B)>0$):
$$
eta(A,B)=fracP(A cap B)P(A) P(B)
$$
so if $eta$ is larger than one, $A$ and $B$ occurs together more often than under independence. Then we can say that $A$ and $B$ are positively related.
But now, using the definition of conditional probability, $fracP(A cap B)P(A) P(B)>1$ is an easy consequence of $P(B mid A) > P(B)$. But $fracP(A cap B)P(A) P(B)$ is completely symmetric in $A$ and $B$ (interchanging all occurrences of the symbol $A$ with $B$ and vice versa) leaves the same formulas, so is also equivalent with $P(A mid B) > P(A)$. That gives the result. So the intuition you ask for is that $eta(A,B)$ is symmetric in $A$ and $B$.
The answer by @gunes gave a practical example, and it is easy to make others the same way.
$endgroup$
To add on the answer by @Dasherman: What can it mean to say that two events are related, or maybe associated or correlated? Maybe we could for a definition compare the joint probability (Assuming $DeclareMathOperatorPmathbbP P(A)>0, P(B)>0$):
$$
eta(A,B)=fracP(A cap B)P(A) P(B)
$$
so if $eta$ is larger than one, $A$ and $B$ occurs together more often than under independence. Then we can say that $A$ and $B$ are positively related.
But now, using the definition of conditional probability, $fracP(A cap B)P(A) P(B)>1$ is an easy consequence of $P(B mid A) > P(B)$. But $fracP(A cap B)P(A) P(B)$ is completely symmetric in $A$ and $B$ (interchanging all occurrences of the symbol $A$ with $B$ and vice versa) leaves the same formulas, so is also equivalent with $P(A mid B) > P(A)$. That gives the result. So the intuition you ask for is that $eta(A,B)$ is symmetric in $A$ and $B$.
The answer by @gunes gave a practical example, and it is easy to make others the same way.
edited 15 hours ago
answered 19 hours ago


kjetil b halvorsenkjetil b halvorsen
32.4k985241
32.4k985241
add a comment |
add a comment |
$begingroup$
I think another mathematical way of putting it may help. Consider the claim in the context of Bayes' rule:
Claim: if $P(B|A)>P(B)$ then $P(A|B) > P(A)$
Bayes' rule:
$$ P(A mid B) = fracP(B mid A) , P(A)P(B) $$
assuming $P(B)$ nonzero. Thus
$$fracB)P(A) = fracP(BP(B)$$
If $P(B|A)>P(B)$, then $fracP(BP(B) > 1$.
Then $fracB)P(A) > 1$, and so $P(A|B) > P(A)$.
This proves the claim and an even stronger conclusion - that the respective proportions of the likelihoods must be equal.
$endgroup$
add a comment |
$begingroup$
I think another mathematical way of putting it may help. Consider the claim in the context of Bayes' rule:
Claim: if $P(B|A)>P(B)$ then $P(A|B) > P(A)$
Bayes' rule:
$$ P(A mid B) = fracP(B mid A) , P(A)P(B) $$
assuming $P(B)$ nonzero. Thus
$$fracB)P(A) = fracP(BP(B)$$
If $P(B|A)>P(B)$, then $fracP(BP(B) > 1$.
Then $fracB)P(A) > 1$, and so $P(A|B) > P(A)$.
This proves the claim and an even stronger conclusion - that the respective proportions of the likelihoods must be equal.
$endgroup$
add a comment |
$begingroup$
I think another mathematical way of putting it may help. Consider the claim in the context of Bayes' rule:
Claim: if $P(B|A)>P(B)$ then $P(A|B) > P(A)$
Bayes' rule:
$$ P(A mid B) = fracP(B mid A) , P(A)P(B) $$
assuming $P(B)$ nonzero. Thus
$$fracB)P(A) = fracP(BP(B)$$
If $P(B|A)>P(B)$, then $fracP(BP(B) > 1$.
Then $fracB)P(A) > 1$, and so $P(A|B) > P(A)$.
This proves the claim and an even stronger conclusion - that the respective proportions of the likelihoods must be equal.
$endgroup$
I think another mathematical way of putting it may help. Consider the claim in the context of Bayes' rule:
Claim: if $P(B|A)>P(B)$ then $P(A|B) > P(A)$
Bayes' rule:
$$ P(A mid B) = fracP(B mid A) , P(A)P(B) $$
assuming $P(B)$ nonzero. Thus
$$fracB)P(A) = fracP(BP(B)$$
If $P(B|A)>P(B)$, then $fracP(BP(B) > 1$.
Then $fracB)P(A) > 1$, and so $P(A|B) > P(A)$.
This proves the claim and an even stronger conclusion - that the respective proportions of the likelihoods must be equal.
edited 12 hours ago
answered 14 hours ago


Aaron HallAaron Hall
251214
251214
add a comment |
add a comment |
$begingroup$
Well, I don't like the word "makes" in the question. That implies some sort of causality and causality usually doesn't reverse.
But you asked for intuition. So, I'd think about some examples, because that seems to spark intuition. Choose one you like:
If a person is a woman, it is more likely that the person voted for a Democrat.
If a person voted for a Democrat, it is more likely that the person is a woman.
If a man is a professional basketball center, it is more likely that he is over 2 meters tall.
If a man is over 2 meters tall, it is more likely that he is a basketball center.
If it is over 40 degrees Celsius, it is more likely that there will be a blackout.
If there has been a blackout, it is more likely that it is over 40 degrees.
And so on.
$endgroup$
2
$begingroup$
That's not about probability. That's about 1 to 1 relationships.
$endgroup$
– Peter Flom♦
16 hours ago
2
$begingroup$
@jww Imagine the statement "if it is raining, the street is wet" (and suppose that's a valid implication for the moment, while the converse is not). Now take a large number of "samples" in different times and places, where you record whether it's raining and whether the street is wet. The street will be wet in more of the samples where it's raining than the samples where it's not; but also, it will be raining in more of the samples where the street is wet than the samples where the street is dry. That's probability.
$endgroup$
– hobbs
14 hours ago
1
$begingroup$
Both phenomena are caused by the same implication; the implication only works one way, but observing the consequent makes it more likely that you're looking at a sample where the antecedent is true.
$endgroup$
– hobbs
14 hours ago
1
$begingroup$
@Barmar Sorry, but that partly demonstrates the correctness of my logic. Because say 36/25,000 is a whole lot higher than 1/150,000,000.
$endgroup$
– Peter Flom♦
9 hours ago
1
$begingroup$
More likely than someone who is less than 2 meters tall.
$endgroup$
– Peter Flom♦
9 hours ago
|
show 4 more comments
$begingroup$
Well, I don't like the word "makes" in the question. That implies some sort of causality and causality usually doesn't reverse.
But you asked for intuition. So, I'd think about some examples, because that seems to spark intuition. Choose one you like:
If a person is a woman, it is more likely that the person voted for a Democrat.
If a person voted for a Democrat, it is more likely that the person is a woman.
If a man is a professional basketball center, it is more likely that he is over 2 meters tall.
If a man is over 2 meters tall, it is more likely that he is a basketball center.
If it is over 40 degrees Celsius, it is more likely that there will be a blackout.
If there has been a blackout, it is more likely that it is over 40 degrees.
And so on.
$endgroup$
2
$begingroup$
That's not about probability. That's about 1 to 1 relationships.
$endgroup$
– Peter Flom♦
16 hours ago
2
$begingroup$
@jww Imagine the statement "if it is raining, the street is wet" (and suppose that's a valid implication for the moment, while the converse is not). Now take a large number of "samples" in different times and places, where you record whether it's raining and whether the street is wet. The street will be wet in more of the samples where it's raining than the samples where it's not; but also, it will be raining in more of the samples where the street is wet than the samples where the street is dry. That's probability.
$endgroup$
– hobbs
14 hours ago
1
$begingroup$
Both phenomena are caused by the same implication; the implication only works one way, but observing the consequent makes it more likely that you're looking at a sample where the antecedent is true.
$endgroup$
– hobbs
14 hours ago
1
$begingroup$
@Barmar Sorry, but that partly demonstrates the correctness of my logic. Because say 36/25,000 is a whole lot higher than 1/150,000,000.
$endgroup$
– Peter Flom♦
9 hours ago
1
$begingroup$
More likely than someone who is less than 2 meters tall.
$endgroup$
– Peter Flom♦
9 hours ago
|
show 4 more comments
$begingroup$
Well, I don't like the word "makes" in the question. That implies some sort of causality and causality usually doesn't reverse.
But you asked for intuition. So, I'd think about some examples, because that seems to spark intuition. Choose one you like:
If a person is a woman, it is more likely that the person voted for a Democrat.
If a person voted for a Democrat, it is more likely that the person is a woman.
If a man is a professional basketball center, it is more likely that he is over 2 meters tall.
If a man is over 2 meters tall, it is more likely that he is a basketball center.
If it is over 40 degrees Celsius, it is more likely that there will be a blackout.
If there has been a blackout, it is more likely that it is over 40 degrees.
And so on.
$endgroup$
Well, I don't like the word "makes" in the question. That implies some sort of causality and causality usually doesn't reverse.
But you asked for intuition. So, I'd think about some examples, because that seems to spark intuition. Choose one you like:
If a person is a woman, it is more likely that the person voted for a Democrat.
If a person voted for a Democrat, it is more likely that the person is a woman.
If a man is a professional basketball center, it is more likely that he is over 2 meters tall.
If a man is over 2 meters tall, it is more likely that he is a basketball center.
If it is over 40 degrees Celsius, it is more likely that there will be a blackout.
If there has been a blackout, it is more likely that it is over 40 degrees.
And so on.
edited 16 hours ago
answered 18 hours ago
Peter Flom♦Peter Flom
77.5k12109218
77.5k12109218
2
$begingroup$
That's not about probability. That's about 1 to 1 relationships.
$endgroup$
– Peter Flom♦
16 hours ago
2
$begingroup$
@jww Imagine the statement "if it is raining, the street is wet" (and suppose that's a valid implication for the moment, while the converse is not). Now take a large number of "samples" in different times and places, where you record whether it's raining and whether the street is wet. The street will be wet in more of the samples where it's raining than the samples where it's not; but also, it will be raining in more of the samples where the street is wet than the samples where the street is dry. That's probability.
$endgroup$
– hobbs
14 hours ago
1
$begingroup$
Both phenomena are caused by the same implication; the implication only works one way, but observing the consequent makes it more likely that you're looking at a sample where the antecedent is true.
$endgroup$
– hobbs
14 hours ago
1
$begingroup$
@Barmar Sorry, but that partly demonstrates the correctness of my logic. Because say 36/25,000 is a whole lot higher than 1/150,000,000.
$endgroup$
– Peter Flom♦
9 hours ago
1
$begingroup$
More likely than someone who is less than 2 meters tall.
$endgroup$
– Peter Flom♦
9 hours ago
|
show 4 more comments
2
$begingroup$
That's not about probability. That's about 1 to 1 relationships.
$endgroup$
– Peter Flom♦
16 hours ago
2
$begingroup$
@jww Imagine the statement "if it is raining, the street is wet" (and suppose that's a valid implication for the moment, while the converse is not). Now take a large number of "samples" in different times and places, where you record whether it's raining and whether the street is wet. The street will be wet in more of the samples where it's raining than the samples where it's not; but also, it will be raining in more of the samples where the street is wet than the samples where the street is dry. That's probability.
$endgroup$
– hobbs
14 hours ago
1
$begingroup$
Both phenomena are caused by the same implication; the implication only works one way, but observing the consequent makes it more likely that you're looking at a sample where the antecedent is true.
$endgroup$
– hobbs
14 hours ago
1
$begingroup$
@Barmar Sorry, but that partly demonstrates the correctness of my logic. Because say 36/25,000 is a whole lot higher than 1/150,000,000.
$endgroup$
– Peter Flom♦
9 hours ago
1
$begingroup$
More likely than someone who is less than 2 meters tall.
$endgroup$
– Peter Flom♦
9 hours ago
2
2
$begingroup$
That's not about probability. That's about 1 to 1 relationships.
$endgroup$
– Peter Flom♦
16 hours ago
$begingroup$
That's not about probability. That's about 1 to 1 relationships.
$endgroup$
– Peter Flom♦
16 hours ago
2
2
$begingroup$
@jww Imagine the statement "if it is raining, the street is wet" (and suppose that's a valid implication for the moment, while the converse is not). Now take a large number of "samples" in different times and places, where you record whether it's raining and whether the street is wet. The street will be wet in more of the samples where it's raining than the samples where it's not; but also, it will be raining in more of the samples where the street is wet than the samples where the street is dry. That's probability.
$endgroup$
– hobbs
14 hours ago
$begingroup$
@jww Imagine the statement "if it is raining, the street is wet" (and suppose that's a valid implication for the moment, while the converse is not). Now take a large number of "samples" in different times and places, where you record whether it's raining and whether the street is wet. The street will be wet in more of the samples where it's raining than the samples where it's not; but also, it will be raining in more of the samples where the street is wet than the samples where the street is dry. That's probability.
$endgroup$
– hobbs
14 hours ago
1
1
$begingroup$
Both phenomena are caused by the same implication; the implication only works one way, but observing the consequent makes it more likely that you're looking at a sample where the antecedent is true.
$endgroup$
– hobbs
14 hours ago
$begingroup$
Both phenomena are caused by the same implication; the implication only works one way, but observing the consequent makes it more likely that you're looking at a sample where the antecedent is true.
$endgroup$
– hobbs
14 hours ago
1
1
$begingroup$
@Barmar Sorry, but that partly demonstrates the correctness of my logic. Because say 36/25,000 is a whole lot higher than 1/150,000,000.
$endgroup$
– Peter Flom♦
9 hours ago
$begingroup$
@Barmar Sorry, but that partly demonstrates the correctness of my logic. Because say 36/25,000 is a whole lot higher than 1/150,000,000.
$endgroup$
– Peter Flom♦
9 hours ago
1
1
$begingroup$
More likely than someone who is less than 2 meters tall.
$endgroup$
– Peter Flom♦
9 hours ago
$begingroup$
More likely than someone who is less than 2 meters tall.
$endgroup$
– Peter Flom♦
9 hours ago
|
show 4 more comments
$begingroup$
By way of intuition, real world examples such as Peter Flom gives are most helpful for some people. The other thing that commonly helps people is pictures. So, to cover most bases, let's have some pictures.
What we have here are two very basic diagrams showing probabilities. The first shows two independent predicates I'll call Red and Plain. It is clear that they are independent because the lines line up. The proportion of plain area that is red is the same as the proportion of stripy area that is red and is also the same as the total proportion that is red.
In the second image, we have non-independent distributions. Specifically, we have moved some of the stripy red area into the plain area without changing the fact that it is red. Clearly then, being red makes being plain more likely.
Meanwhile, have a look at the plain side of that image. Clearly the proportion of the plain region that is red is greater than the proportion of the whole image that is red. That is because the plain region has been given a bunch more area and all of it is red.
So, red makes plain more likely, and plain makes red more likely.
What's actually happening here? A is evidence for B (that is, A makes B more likely) when the area that contains both A and B is bigger than would be predicted if they were independent. Because the intersection between A and B is the same as the intersection between B and A, that also implies that B is evidence for A.
One note of caution: although the argument above seems very symmetrical, it may not be the case that the strength of evidence in both directions is equal. For example, consider this third image.
Here the same thing has happened: plain red has eaten up territory previously belonging to stripy red. In fact, it has completely finished the job!
Note that the point being red outright guarantees plainness because there are no stripy red regions left. However a point being plain has not guaranteed redness, because there are still green regions left. Nevertheless, a point in the box being plain increases the chance that it is red, and a point being red increases the chance that it is plain. Both directions imply more likely, just not by the same amount.
New contributor
Josiah is a new contributor to this site. Take care in asking for clarification, commenting, and answering.
Check out our Code of Conduct.
$endgroup$
add a comment |
$begingroup$
By way of intuition, real world examples such as Peter Flom gives are most helpful for some people. The other thing that commonly helps people is pictures. So, to cover most bases, let's have some pictures.
What we have here are two very basic diagrams showing probabilities. The first shows two independent predicates I'll call Red and Plain. It is clear that they are independent because the lines line up. The proportion of plain area that is red is the same as the proportion of stripy area that is red and is also the same as the total proportion that is red.
In the second image, we have non-independent distributions. Specifically, we have moved some of the stripy red area into the plain area without changing the fact that it is red. Clearly then, being red makes being plain more likely.
Meanwhile, have a look at the plain side of that image. Clearly the proportion of the plain region that is red is greater than the proportion of the whole image that is red. That is because the plain region has been given a bunch more area and all of it is red.
So, red makes plain more likely, and plain makes red more likely.
What's actually happening here? A is evidence for B (that is, A makes B more likely) when the area that contains both A and B is bigger than would be predicted if they were independent. Because the intersection between A and B is the same as the intersection between B and A, that also implies that B is evidence for A.
One note of caution: although the argument above seems very symmetrical, it may not be the case that the strength of evidence in both directions is equal. For example, consider this third image.
Here the same thing has happened: plain red has eaten up territory previously belonging to stripy red. In fact, it has completely finished the job!
Note that the point being red outright guarantees plainness because there are no stripy red regions left. However a point being plain has not guaranteed redness, because there are still green regions left. Nevertheless, a point in the box being plain increases the chance that it is red, and a point being red increases the chance that it is plain. Both directions imply more likely, just not by the same amount.
New contributor
Josiah is a new contributor to this site. Take care in asking for clarification, commenting, and answering.
Check out our Code of Conduct.
$endgroup$
add a comment |
$begingroup$
By way of intuition, real world examples such as Peter Flom gives are most helpful for some people. The other thing that commonly helps people is pictures. So, to cover most bases, let's have some pictures.
What we have here are two very basic diagrams showing probabilities. The first shows two independent predicates I'll call Red and Plain. It is clear that they are independent because the lines line up. The proportion of plain area that is red is the same as the proportion of stripy area that is red and is also the same as the total proportion that is red.
In the second image, we have non-independent distributions. Specifically, we have moved some of the stripy red area into the plain area without changing the fact that it is red. Clearly then, being red makes being plain more likely.
Meanwhile, have a look at the plain side of that image. Clearly the proportion of the plain region that is red is greater than the proportion of the whole image that is red. That is because the plain region has been given a bunch more area and all of it is red.
So, red makes plain more likely, and plain makes red more likely.
What's actually happening here? A is evidence for B (that is, A makes B more likely) when the area that contains both A and B is bigger than would be predicted if they were independent. Because the intersection between A and B is the same as the intersection between B and A, that also implies that B is evidence for A.
One note of caution: although the argument above seems very symmetrical, it may not be the case that the strength of evidence in both directions is equal. For example, consider this third image.
Here the same thing has happened: plain red has eaten up territory previously belonging to stripy red. In fact, it has completely finished the job!
Note that the point being red outright guarantees plainness because there are no stripy red regions left. However a point being plain has not guaranteed redness, because there are still green regions left. Nevertheless, a point in the box being plain increases the chance that it is red, and a point being red increases the chance that it is plain. Both directions imply more likely, just not by the same amount.
New contributor
Josiah is a new contributor to this site. Take care in asking for clarification, commenting, and answering.
Check out our Code of Conduct.
$endgroup$
By way of intuition, real world examples such as Peter Flom gives are most helpful for some people. The other thing that commonly helps people is pictures. So, to cover most bases, let's have some pictures.
What we have here are two very basic diagrams showing probabilities. The first shows two independent predicates I'll call Red and Plain. It is clear that they are independent because the lines line up. The proportion of plain area that is red is the same as the proportion of stripy area that is red and is also the same as the total proportion that is red.
In the second image, we have non-independent distributions. Specifically, we have moved some of the stripy red area into the plain area without changing the fact that it is red. Clearly then, being red makes being plain more likely.
Meanwhile, have a look at the plain side of that image. Clearly the proportion of the plain region that is red is greater than the proportion of the whole image that is red. That is because the plain region has been given a bunch more area and all of it is red.
So, red makes plain more likely, and plain makes red more likely.
What's actually happening here? A is evidence for B (that is, A makes B more likely) when the area that contains both A and B is bigger than would be predicted if they were independent. Because the intersection between A and B is the same as the intersection between B and A, that also implies that B is evidence for A.
One note of caution: although the argument above seems very symmetrical, it may not be the case that the strength of evidence in both directions is equal. For example, consider this third image.
Here the same thing has happened: plain red has eaten up territory previously belonging to stripy red. In fact, it has completely finished the job!
Note that the point being red outright guarantees plainness because there are no stripy red regions left. However a point being plain has not guaranteed redness, because there are still green regions left. Nevertheless, a point in the box being plain increases the chance that it is red, and a point being red increases the chance that it is plain. Both directions imply more likely, just not by the same amount.
New contributor
Josiah is a new contributor to this site. Take care in asking for clarification, commenting, and answering.
Check out our Code of Conduct.
New contributor
Josiah is a new contributor to this site. Take care in asking for clarification, commenting, and answering.
Check out our Code of Conduct.
answered 10 hours ago
JosiahJosiah
1312
1312
New contributor
Josiah is a new contributor to this site. Take care in asking for clarification, commenting, and answering.
Check out our Code of Conduct.
New contributor
Josiah is a new contributor to this site. Take care in asking for clarification, commenting, and answering.
Check out our Code of Conduct.
Josiah is a new contributor to this site. Take care in asking for clarification, commenting, and answering.
Check out our Code of Conduct.
add a comment |
add a comment |
$begingroup$
If A makes B more likely, this means the events are somehow related. This relation works both ways.
If A makes B more likely, this means that A and B tend to happen together. This then means that B also makes A more likely.
$endgroup$
1
$begingroup$
This perhaps could use some expansion? Without a definition of related it is a bit empty.
$endgroup$
– mdewey
19 hours ago
2
$begingroup$
I was trying to stay away from anything rigorous, since OP asked for an intuitive explanation. You are right that it is quite empty as it is now, but I'm not sure how to expand it in an intuitive way. I have added an attempt.
$endgroup$
– Dasherman
18 hours ago
add a comment |
$begingroup$
If A makes B more likely, this means the events are somehow related. This relation works both ways.
If A makes B more likely, this means that A and B tend to happen together. This then means that B also makes A more likely.
$endgroup$
1
$begingroup$
This perhaps could use some expansion? Without a definition of related it is a bit empty.
$endgroup$
– mdewey
19 hours ago
2
$begingroup$
I was trying to stay away from anything rigorous, since OP asked for an intuitive explanation. You are right that it is quite empty as it is now, but I'm not sure how to expand it in an intuitive way. I have added an attempt.
$endgroup$
– Dasherman
18 hours ago
add a comment |
$begingroup$
If A makes B more likely, this means the events are somehow related. This relation works both ways.
If A makes B more likely, this means that A and B tend to happen together. This then means that B also makes A more likely.
$endgroup$
If A makes B more likely, this means the events are somehow related. This relation works both ways.
If A makes B more likely, this means that A and B tend to happen together. This then means that B also makes A more likely.
edited 18 hours ago
answered 21 hours ago
DashermanDasherman
20116
20116
1
$begingroup$
This perhaps could use some expansion? Without a definition of related it is a bit empty.
$endgroup$
– mdewey
19 hours ago
2
$begingroup$
I was trying to stay away from anything rigorous, since OP asked for an intuitive explanation. You are right that it is quite empty as it is now, but I'm not sure how to expand it in an intuitive way. I have added an attempt.
$endgroup$
– Dasherman
18 hours ago
add a comment |
1
$begingroup$
This perhaps could use some expansion? Without a definition of related it is a bit empty.
$endgroup$
– mdewey
19 hours ago
2
$begingroup$
I was trying to stay away from anything rigorous, since OP asked for an intuitive explanation. You are right that it is quite empty as it is now, but I'm not sure how to expand it in an intuitive way. I have added an attempt.
$endgroup$
– Dasherman
18 hours ago
1
1
$begingroup$
This perhaps could use some expansion? Without a definition of related it is a bit empty.
$endgroup$
– mdewey
19 hours ago
$begingroup$
This perhaps could use some expansion? Without a definition of related it is a bit empty.
$endgroup$
– mdewey
19 hours ago
2
2
$begingroup$
I was trying to stay away from anything rigorous, since OP asked for an intuitive explanation. You are right that it is quite empty as it is now, but I'm not sure how to expand it in an intuitive way. I have added an attempt.
$endgroup$
– Dasherman
18 hours ago
$begingroup$
I was trying to stay away from anything rigorous, since OP asked for an intuitive explanation. You are right that it is quite empty as it is now, but I'm not sure how to expand it in an intuitive way. I have added an attempt.
$endgroup$
– Dasherman
18 hours ago
add a comment |
$begingroup$
If A makes B more likely, A has crucial information that B can infer about itself. Despite the fact that it might not contribute the same amount, that information is not lost the other way around. Eventually, we have two events that their occurrence support each other. I can’t seem to imagine a scenario where occurrence of A increases the likelihood of B, and occurrence of B decreases the likelihood of A. For example, if it rains, the floor will be wet with high probability, and if the floor is wet, it doesn’t mean that it rained but it doesn’t decrease the chances.
$endgroup$
add a comment |
$begingroup$
If A makes B more likely, A has crucial information that B can infer about itself. Despite the fact that it might not contribute the same amount, that information is not lost the other way around. Eventually, we have two events that their occurrence support each other. I can’t seem to imagine a scenario where occurrence of A increases the likelihood of B, and occurrence of B decreases the likelihood of A. For example, if it rains, the floor will be wet with high probability, and if the floor is wet, it doesn’t mean that it rained but it doesn’t decrease the chances.
$endgroup$
add a comment |
$begingroup$
If A makes B more likely, A has crucial information that B can infer about itself. Despite the fact that it might not contribute the same amount, that information is not lost the other way around. Eventually, we have two events that their occurrence support each other. I can’t seem to imagine a scenario where occurrence of A increases the likelihood of B, and occurrence of B decreases the likelihood of A. For example, if it rains, the floor will be wet with high probability, and if the floor is wet, it doesn’t mean that it rained but it doesn’t decrease the chances.
$endgroup$
If A makes B more likely, A has crucial information that B can infer about itself. Despite the fact that it might not contribute the same amount, that information is not lost the other way around. Eventually, we have two events that their occurrence support each other. I can’t seem to imagine a scenario where occurrence of A increases the likelihood of B, and occurrence of B decreases the likelihood of A. For example, if it rains, the floor will be wet with high probability, and if the floor is wet, it doesn’t mean that it rained but it doesn’t decrease the chances.
edited 16 hours ago
answered 23 hours ago
gunesgunes
7,5311316
7,5311316
add a comment |
add a comment |
$begingroup$
You are told that Sam is a woman and Kim is a man, and one of the two wears make-up and the other does not. Who of them would you guess wears make-up?
You are told that Sam wears make-up and Kim doesn't, and one of the two is a man and one is a woman. Who would you guess is the woman?
$endgroup$
$begingroup$
It is not so straightforward to connect this to the original problem. What exactly is event A and what is event B? Here it seems more like some comparison of probabilities. Event A is 'x is a women' (not A is the event 'x is a man'). And event B is 'x wears makeup'. But now we suddenly have a Sam and a Kim, where does that come from and should we use anything of information about the subjective masculinity or femininity of their names?
$endgroup$
– Martijn Weterings
11 hours ago
add a comment |
$begingroup$
You are told that Sam is a woman and Kim is a man, and one of the two wears make-up and the other does not. Who of them would you guess wears make-up?
You are told that Sam wears make-up and Kim doesn't, and one of the two is a man and one is a woman. Who would you guess is the woman?
$endgroup$
$begingroup$
It is not so straightforward to connect this to the original problem. What exactly is event A and what is event B? Here it seems more like some comparison of probabilities. Event A is 'x is a women' (not A is the event 'x is a man'). And event B is 'x wears makeup'. But now we suddenly have a Sam and a Kim, where does that come from and should we use anything of information about the subjective masculinity or femininity of their names?
$endgroup$
– Martijn Weterings
11 hours ago
add a comment |
$begingroup$
You are told that Sam is a woman and Kim is a man, and one of the two wears make-up and the other does not. Who of them would you guess wears make-up?
You are told that Sam wears make-up and Kim doesn't, and one of the two is a man and one is a woman. Who would you guess is the woman?
$endgroup$
You are told that Sam is a woman and Kim is a man, and one of the two wears make-up and the other does not. Who of them would you guess wears make-up?
You are told that Sam wears make-up and Kim doesn't, and one of the two is a man and one is a woman. Who would you guess is the woman?
answered 14 hours ago


Hagen von EitzenHagen von Eitzen
1413
1413
$begingroup$
It is not so straightforward to connect this to the original problem. What exactly is event A and what is event B? Here it seems more like some comparison of probabilities. Event A is 'x is a women' (not A is the event 'x is a man'). And event B is 'x wears makeup'. But now we suddenly have a Sam and a Kim, where does that come from and should we use anything of information about the subjective masculinity or femininity of their names?
$endgroup$
– Martijn Weterings
11 hours ago
add a comment |
$begingroup$
It is not so straightforward to connect this to the original problem. What exactly is event A and what is event B? Here it seems more like some comparison of probabilities. Event A is 'x is a women' (not A is the event 'x is a man'). And event B is 'x wears makeup'. But now we suddenly have a Sam and a Kim, where does that come from and should we use anything of information about the subjective masculinity or femininity of their names?
$endgroup$
– Martijn Weterings
11 hours ago
$begingroup$
It is not so straightforward to connect this to the original problem. What exactly is event A and what is event B? Here it seems more like some comparison of probabilities. Event A is 'x is a women' (not A is the event 'x is a man'). And event B is 'x wears makeup'. But now we suddenly have a Sam and a Kim, where does that come from and should we use anything of information about the subjective masculinity or femininity of their names?
$endgroup$
– Martijn Weterings
11 hours ago
$begingroup$
It is not so straightforward to connect this to the original problem. What exactly is event A and what is event B? Here it seems more like some comparison of probabilities. Event A is 'x is a women' (not A is the event 'x is a man'). And event B is 'x wears makeup'. But now we suddenly have a Sam and a Kim, where does that come from and should we use anything of information about the subjective masculinity or femininity of their names?
$endgroup$
– Martijn Weterings
11 hours ago
add a comment |
$begingroup$
You can make the math more intuitive by imagining a contingency table.
$beginarraycc
beginarraycc
&& A & lnot A & \
&a+b+c+d & a+c & b+d \hline
B& a+b& a & b \
lnot B & c+d& c & d \
endarray
endarray$
When $A$ and $B$ are independent then the joint probabilities are products of the marginal probabilities $$beginarraycc
beginarraycc
&& A & lnot A & \
&1 & x & 1-x \hline
B& y& a=xy & b=(1-x)y \
lnot B & 1-y& c=x (1-y) & d=(1-x)(1-y)\
endarray
endarray$$ In such case you would have similar marginal and conditional probabilities, e.g. $P (A) = P (A|B) $ and $P (B)=P (B|A) $.When there is no independence then you could see this as leaving the parameters $a,b,c,d $ the same (as products of the margins) but with just an adjustment by $pm z $ $$beginarraycc
beginarraycc
&& A & lnot A & \
&1 & x & 1-x \hline
B& y& a+z & b-z \
lnot B & 1-y& c-z & d+z\
endarray
endarray$$You could see this $z$ as breaking the equality of the marginal and conditional probabilities or breaking the relationship for the joint probabilities being products of the marginal probabilities.
Now, from this point of view (of breaking these equalities) you can see that this breaking happens in two ways both for $P(A|B) neq P(A)$ and $P(B|A) neq P(B)$. And the inequality will be for both cases $>$ when $z$ is positive and $<$ when $z $ is negative.
So you could see the connection $P(A|B) > P(A)$ then $P(B|A) > P(B)$ via the joint probability $P(B,A) > P (A) P (B) $.
If A and B often happen together (joint probability is higher then product of marginal probabilities) then observing the one will make the (conditional) probability of the other higher.
$endgroup$
add a comment |
$begingroup$
You can make the math more intuitive by imagining a contingency table.
$beginarraycc
beginarraycc
&& A & lnot A & \
&a+b+c+d & a+c & b+d \hline
B& a+b& a & b \
lnot B & c+d& c & d \
endarray
endarray$
When $A$ and $B$ are independent then the joint probabilities are products of the marginal probabilities $$beginarraycc
beginarraycc
&& A & lnot A & \
&1 & x & 1-x \hline
B& y& a=xy & b=(1-x)y \
lnot B & 1-y& c=x (1-y) & d=(1-x)(1-y)\
endarray
endarray$$ In such case you would have similar marginal and conditional probabilities, e.g. $P (A) = P (A|B) $ and $P (B)=P (B|A) $.When there is no independence then you could see this as leaving the parameters $a,b,c,d $ the same (as products of the margins) but with just an adjustment by $pm z $ $$beginarraycc
beginarraycc
&& A & lnot A & \
&1 & x & 1-x \hline
B& y& a+z & b-z \
lnot B & 1-y& c-z & d+z\
endarray
endarray$$You could see this $z$ as breaking the equality of the marginal and conditional probabilities or breaking the relationship for the joint probabilities being products of the marginal probabilities.
Now, from this point of view (of breaking these equalities) you can see that this breaking happens in two ways both for $P(A|B) neq P(A)$ and $P(B|A) neq P(B)$. And the inequality will be for both cases $>$ when $z$ is positive and $<$ when $z $ is negative.
So you could see the connection $P(A|B) > P(A)$ then $P(B|A) > P(B)$ via the joint probability $P(B,A) > P (A) P (B) $.
If A and B often happen together (joint probability is higher then product of marginal probabilities) then observing the one will make the (conditional) probability of the other higher.
$endgroup$
add a comment |
$begingroup$
You can make the math more intuitive by imagining a contingency table.
$beginarraycc
beginarraycc
&& A & lnot A & \
&a+b+c+d & a+c & b+d \hline
B& a+b& a & b \
lnot B & c+d& c & d \
endarray
endarray$
When $A$ and $B$ are independent then the joint probabilities are products of the marginal probabilities $$beginarraycc
beginarraycc
&& A & lnot A & \
&1 & x & 1-x \hline
B& y& a=xy & b=(1-x)y \
lnot B & 1-y& c=x (1-y) & d=(1-x)(1-y)\
endarray
endarray$$ In such case you would have similar marginal and conditional probabilities, e.g. $P (A) = P (A|B) $ and $P (B)=P (B|A) $.When there is no independence then you could see this as leaving the parameters $a,b,c,d $ the same (as products of the margins) but with just an adjustment by $pm z $ $$beginarraycc
beginarraycc
&& A & lnot A & \
&1 & x & 1-x \hline
B& y& a+z & b-z \
lnot B & 1-y& c-z & d+z\
endarray
endarray$$You could see this $z$ as breaking the equality of the marginal and conditional probabilities or breaking the relationship for the joint probabilities being products of the marginal probabilities.
Now, from this point of view (of breaking these equalities) you can see that this breaking happens in two ways both for $P(A|B) neq P(A)$ and $P(B|A) neq P(B)$. And the inequality will be for both cases $>$ when $z$ is positive and $<$ when $z $ is negative.
So you could see the connection $P(A|B) > P(A)$ then $P(B|A) > P(B)$ via the joint probability $P(B,A) > P (A) P (B) $.
If A and B often happen together (joint probability is higher then product of marginal probabilities) then observing the one will make the (conditional) probability of the other higher.
$endgroup$
You can make the math more intuitive by imagining a contingency table.
$beginarraycc
beginarraycc
&& A & lnot A & \
&a+b+c+d & a+c & b+d \hline
B& a+b& a & b \
lnot B & c+d& c & d \
endarray
endarray$
When $A$ and $B$ are independent then the joint probabilities are products of the marginal probabilities $$beginarraycc
beginarraycc
&& A & lnot A & \
&1 & x & 1-x \hline
B& y& a=xy & b=(1-x)y \
lnot B & 1-y& c=x (1-y) & d=(1-x)(1-y)\
endarray
endarray$$ In such case you would have similar marginal and conditional probabilities, e.g. $P (A) = P (A|B) $ and $P (B)=P (B|A) $.When there is no independence then you could see this as leaving the parameters $a,b,c,d $ the same (as products of the margins) but with just an adjustment by $pm z $ $$beginarraycc
beginarraycc
&& A & lnot A & \
&1 & x & 1-x \hline
B& y& a+z & b-z \
lnot B & 1-y& c-z & d+z\
endarray
endarray$$You could see this $z$ as breaking the equality of the marginal and conditional probabilities or breaking the relationship for the joint probabilities being products of the marginal probabilities.
Now, from this point of view (of breaking these equalities) you can see that this breaking happens in two ways both for $P(A|B) neq P(A)$ and $P(B|A) neq P(B)$. And the inequality will be for both cases $>$ when $z$ is positive and $<$ when $z $ is negative.
So you could see the connection $P(A|B) > P(A)$ then $P(B|A) > P(B)$ via the joint probability $P(B,A) > P (A) P (B) $.
If A and B often happen together (joint probability is higher then product of marginal probabilities) then observing the one will make the (conditional) probability of the other higher.
edited 11 hours ago
answered 12 hours ago
Martijn WeteringsMartijn Weterings
14.8k2064
14.8k2064
add a comment |
add a comment |
$begingroup$
Suppose we denote the posterior-to-prior probability ratio of an event as:
$$Delta(A|B) equiv fracB)mathbbP(A)$$
Then an alternative expression of Bayes' theorem (see this related post) is:
$$Delta(A|B)
= fracB)mathbbP(A)
= fracmathbbP(A cap B)mathbbP(A) mathbbP(B)
= fracA)mathbbP(B)
= Delta(B|A).$$
The posterior-to-prior probability ratio tells us whether the argument event is made more or less likely by the occurrence of the conditioning event (and how much more or less likely). The above form of Bayes' theorem shows use that posterior-to-prior probability ratio is symmetric in the variables.$^dagger$ For example, if observing $B$ makes $A$ more likely than it was a priori, then observing $A$ makes $B$ more likely than it was a priori.
$^dagger$ Note that this is a probability rule, and so it should not be interpreted causally. This symmetry is true in a probabilistic sense for passive observation ---however, it is not true if you intervene in the system to change $A$ or $B$. In that latter case you would need to use causal operations (e.g., the $textdo$ operator) to find the effect of the change in the conditioning variable.
$endgroup$
add a comment |
$begingroup$
Suppose we denote the posterior-to-prior probability ratio of an event as:
$$Delta(A|B) equiv fracB)mathbbP(A)$$
Then an alternative expression of Bayes' theorem (see this related post) is:
$$Delta(A|B)
= fracB)mathbbP(A)
= fracmathbbP(A cap B)mathbbP(A) mathbbP(B)
= fracA)mathbbP(B)
= Delta(B|A).$$
The posterior-to-prior probability ratio tells us whether the argument event is made more or less likely by the occurrence of the conditioning event (and how much more or less likely). The above form of Bayes' theorem shows use that posterior-to-prior probability ratio is symmetric in the variables.$^dagger$ For example, if observing $B$ makes $A$ more likely than it was a priori, then observing $A$ makes $B$ more likely than it was a priori.
$^dagger$ Note that this is a probability rule, and so it should not be interpreted causally. This symmetry is true in a probabilistic sense for passive observation ---however, it is not true if you intervene in the system to change $A$ or $B$. In that latter case you would need to use causal operations (e.g., the $textdo$ operator) to find the effect of the change in the conditioning variable.
$endgroup$
add a comment |
$begingroup$
Suppose we denote the posterior-to-prior probability ratio of an event as:
$$Delta(A|B) equiv fracB)mathbbP(A)$$
Then an alternative expression of Bayes' theorem (see this related post) is:
$$Delta(A|B)
= fracB)mathbbP(A)
= fracmathbbP(A cap B)mathbbP(A) mathbbP(B)
= fracA)mathbbP(B)
= Delta(B|A).$$
The posterior-to-prior probability ratio tells us whether the argument event is made more or less likely by the occurrence of the conditioning event (and how much more or less likely). The above form of Bayes' theorem shows use that posterior-to-prior probability ratio is symmetric in the variables.$^dagger$ For example, if observing $B$ makes $A$ more likely than it was a priori, then observing $A$ makes $B$ more likely than it was a priori.
$^dagger$ Note that this is a probability rule, and so it should not be interpreted causally. This symmetry is true in a probabilistic sense for passive observation ---however, it is not true if you intervene in the system to change $A$ or $B$. In that latter case you would need to use causal operations (e.g., the $textdo$ operator) to find the effect of the change in the conditioning variable.
$endgroup$
Suppose we denote the posterior-to-prior probability ratio of an event as:
$$Delta(A|B) equiv fracB)mathbbP(A)$$
Then an alternative expression of Bayes' theorem (see this related post) is:
$$Delta(A|B)
= fracB)mathbbP(A)
= fracmathbbP(A cap B)mathbbP(A) mathbbP(B)
= fracA)mathbbP(B)
= Delta(B|A).$$
The posterior-to-prior probability ratio tells us whether the argument event is made more or less likely by the occurrence of the conditioning event (and how much more or less likely). The above form of Bayes' theorem shows use that posterior-to-prior probability ratio is symmetric in the variables.$^dagger$ For example, if observing $B$ makes $A$ more likely than it was a priori, then observing $A$ makes $B$ more likely than it was a priori.
$^dagger$ Note that this is a probability rule, and so it should not be interpreted causally. This symmetry is true in a probabilistic sense for passive observation ---however, it is not true if you intervene in the system to change $A$ or $B$. In that latter case you would need to use causal operations (e.g., the $textdo$ operator) to find the effect of the change in the conditioning variable.
answered 5 hours ago


BenBen
28.3k233128
28.3k233128
add a comment |
add a comment |
$begingroup$
There is a confusion here between causation and correlation. So I'll give you an example where the exact opposite happens.
Some people are rich, some are poor. Some poor people are given benefits, which makes them less poor. But people who get benefits are still more likely to be poor, even with benefits.
If you are given benefits, that makes it more likely that you can afford cinema tickets. ("Makes it more likely" meaning causality). But if you can afford cinema tickets, that makes it less likely that you are among the people who are poor enough to get benefits, so if you can afford cinema tickets, you are less likely to get benefits.
$endgroup$
4
$begingroup$
This isn't an answer to the question. Interesting, but not an answer. In fact, it's talking about a different scenario; the reason the opposite happens is that it's using two different metrics that are named similarly (poor without benefits v.s. poor with benefits) and as such is a completely different scenario.
$endgroup$
– wizzwizz4
16 hours ago
add a comment |
$begingroup$
There is a confusion here between causation and correlation. So I'll give you an example where the exact opposite happens.
Some people are rich, some are poor. Some poor people are given benefits, which makes them less poor. But people who get benefits are still more likely to be poor, even with benefits.
If you are given benefits, that makes it more likely that you can afford cinema tickets. ("Makes it more likely" meaning causality). But if you can afford cinema tickets, that makes it less likely that you are among the people who are poor enough to get benefits, so if you can afford cinema tickets, you are less likely to get benefits.
$endgroup$
4
$begingroup$
This isn't an answer to the question. Interesting, but not an answer. In fact, it's talking about a different scenario; the reason the opposite happens is that it's using two different metrics that are named similarly (poor without benefits v.s. poor with benefits) and as such is a completely different scenario.
$endgroup$
– wizzwizz4
16 hours ago
add a comment |
$begingroup$
There is a confusion here between causation and correlation. So I'll give you an example where the exact opposite happens.
Some people are rich, some are poor. Some poor people are given benefits, which makes them less poor. But people who get benefits are still more likely to be poor, even with benefits.
If you are given benefits, that makes it more likely that you can afford cinema tickets. ("Makes it more likely" meaning causality). But if you can afford cinema tickets, that makes it less likely that you are among the people who are poor enough to get benefits, so if you can afford cinema tickets, you are less likely to get benefits.
$endgroup$
There is a confusion here between causation and correlation. So I'll give you an example where the exact opposite happens.
Some people are rich, some are poor. Some poor people are given benefits, which makes them less poor. But people who get benefits are still more likely to be poor, even with benefits.
If you are given benefits, that makes it more likely that you can afford cinema tickets. ("Makes it more likely" meaning causality). But if you can afford cinema tickets, that makes it less likely that you are among the people who are poor enough to get benefits, so if you can afford cinema tickets, you are less likely to get benefits.
answered 16 hours ago
gnasher729gnasher729
48133
48133
4
$begingroup$
This isn't an answer to the question. Interesting, but not an answer. In fact, it's talking about a different scenario; the reason the opposite happens is that it's using two different metrics that are named similarly (poor without benefits v.s. poor with benefits) and as such is a completely different scenario.
$endgroup$
– wizzwizz4
16 hours ago
add a comment |
4
$begingroup$
This isn't an answer to the question. Interesting, but not an answer. In fact, it's talking about a different scenario; the reason the opposite happens is that it's using two different metrics that are named similarly (poor without benefits v.s. poor with benefits) and as such is a completely different scenario.
$endgroup$
– wizzwizz4
16 hours ago
4
4
$begingroup$
This isn't an answer to the question. Interesting, but not an answer. In fact, it's talking about a different scenario; the reason the opposite happens is that it's using two different metrics that are named similarly (poor without benefits v.s. poor with benefits) and as such is a completely different scenario.
$endgroup$
– wizzwizz4
16 hours ago
$begingroup$
This isn't an answer to the question. Interesting, but not an answer. In fact, it's talking about a different scenario; the reason the opposite happens is that it's using two different metrics that are named similarly (poor without benefits v.s. poor with benefits) and as such is a completely different scenario.
$endgroup$
– wizzwizz4
16 hours ago
add a comment |
Thanks for contributing an answer to Cross Validated!
- Please be sure to answer the question. Provide details and share your research!
But avoid …
- Asking for help, clarification, or responding to other answers.
- Making statements based on opinion; back them up with references or personal experience.
Use MathJax to format equations. MathJax reference.
To learn more, see our tips on writing great answers.
Sign up or log in
StackExchange.ready(function ()
StackExchange.helpers.onClickDraftSave('#login-link');
);
Sign up using Google
Sign up using Facebook
Sign up using Email and Password
Post as a guest
Required, but never shown
StackExchange.ready(
function ()
StackExchange.openid.initPostLogin('.new-post-login', 'https%3a%2f%2fstats.stackexchange.com%2fquestions%2f402935%2fif-a-makes-b-more-likely-then-b-makes-a-more-likely%23new-answer', 'question_page');
);
Post as a guest
Required, but never shown
Sign up or log in
StackExchange.ready(function ()
StackExchange.helpers.onClickDraftSave('#login-link');
);
Sign up using Google
Sign up using Facebook
Sign up using Email and Password
Post as a guest
Required, but never shown
Sign up or log in
StackExchange.ready(function ()
StackExchange.helpers.onClickDraftSave('#login-link');
);
Sign up using Google
Sign up using Facebook
Sign up using Email and Password
Post as a guest
Required, but never shown
Sign up or log in
StackExchange.ready(function ()
StackExchange.helpers.onClickDraftSave('#login-link');
);
Sign up using Google
Sign up using Facebook
Sign up using Email and Password
Sign up using Google
Sign up using Facebook
Sign up using Email and Password
Post as a guest
Required, but never shown
Required, but never shown
Required, but never shown
Required, but never shown
Required, but never shown
Required, but never shown
Required, but never shown
Required, but never shown
Required, but never shown
-association-measure, conditional-probability, inference, intuition, probabilityeS,fmOYpLMZYP OrN91hH jofTk5Azebsyd7Cz5SiakGXVFQ0U5